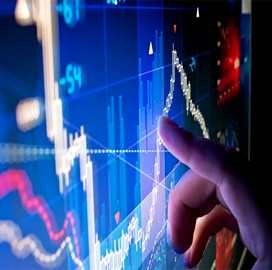
ARL, USC Discover New Cybersecurity Technique for Improved Soldier Graph Analytics
Researchers from the Army Research Laboratory and the University of Southern California have come up with a new cybersecurity technique that is expected to improve soldier graph analytics.
The novel design framework, called GraphSAINT, parallelizes training on the Graph Neural Network, a fundamental aspect for deep learning on graphs, which ARL researcher Rajgopal Kannan considers “analogous to convolutional neural networks that are ubiquitous in Army machine learning applications for vision and image processing such as in target detection and object recognition.”
The researchers developed GraphSAINT, or the Graph SAmpling Based INductive Learning Method, in such a way that would lower the memory and computational requirements of graph machine learning without compromising accuracy, Kannan said, enabling deeper models and faster learning on a larger scale, the Army reported.
With the use of GraphSAINT, the Army can exploit its vast database of graph-based information, leading to the transmission of actionable intelligence for soldiers, Kanna explained.
Overall, the GraphSAINT is envisioned to facilitate enhanced situational awareness, enable cybersecurity applications for threat detection and prevention, as well as for human activity recognition and motion detection.
The research team’s advancements in graph inductive learning also gives soldiers the ability to make inferences on completely unknown graphs.
According to Kannan, the said advantage opens up opportunities for “a machine learning network trained on a known graph of adversary communication patterns.” The edge provided by the learning model extends not only to inferring malicious enemy activities, Kannan said, as soldiers could deny them en route to achieving area superiority and dominance relative to command, control, computers, communications, cyber, intelligence, surveillance and reconnaissance.
However, Kannan said the fulfillment of the aforementioned advantages hinges on the speed, accuracy and scaled approach to both graph training and inference. Currently, the research team is working on beating state-of-the-art Graph Neural Networks in both training time and accuracy on large graph datasets such as Reddit, Amazon, Yelp and Flickr.
Moving forward, Kannan said the research team will work on leveraging the Army’s expertise in accelerating graph algorithms and developing an optimized distributed graph training system dedicated to enable deep learning on larger graphs. The team is also keen on building a complete graph processing system consisting of a querying front-end and an extended GraphSAINT based back-end to support Army modernization priority applications.
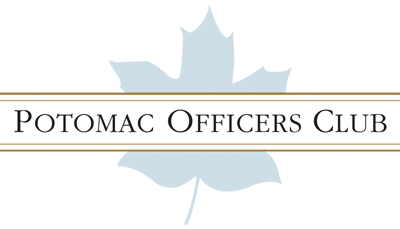
Category: Future Trends