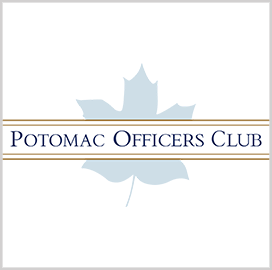
Machine learning
Argonne National Lab Uses AI to Improve Airplane Parts Manufacturing Process
Researchers at the Argonne National Laboratory have turned to artificial intelligence and machine learning to improve the airplane manufacturing process.
The researchers are specifically applying AI to friction stir welding, the process of softening two workpieces without reaching the materials’ melting point, according to friction stir welding tool maker Mazak MegaStir.
The use of machine learning reduces the number of experiments and time-consuming simulations that need to be performed to obtain information on the welding process, ANL said Monday.
The AI-powered approach, which ANL calls DeepHyper, is designed to automate the design and development of machine learning-based predictive models.
ANL computer scientist Prasanna Balaprakash, a lead developer on DeepHyper, said the technology provides the ability to automatically identify the best manufacturing configurations out of a set of a billion possible settings.
“If you’re trying to brew the best cup of coffee, you can spend several hours fiddling with the many settings on the best machines,” Balaprakash said, adding that DeepHyper is designed to eliminate such an issue in the manufacturing of plane parts.
He noted that DeepHyper’s purpose is not to find the single best predictive but to generate a large enough number of accurate models that, when combined together, account for uncertainties in the predictions.
Balaprakash and his colleagues developed DeepHyper as part of the Probabilistic Machine Learning for Rapid Large-Scale and High-Rate Aerostructure Manufacturing project, a collaboration among ANL, GE Research, Edison Welding Institute and GKN Aerospace.
ANL said the effort is funded by the Department of Energy’s Advanced Manufacturing Office.
Category: Federal Civilian